AI vs Machine Learning
We always hear terms like AI, machine learning, and similar terms, but, there’s still a lot of confusion regarding AI and what exactly is machine learning. We often make the mistake of referring to these terms as synonyms, discrete, or parallel advancements. Others are taking advantage of the trend to the hype, excite and increase sales and revenue. There are few basic differences between the two, however. AI is a technology that enables a machine to simulate human behavior. This piece will give you in-depth knowledge about AI vs Machine Learning.
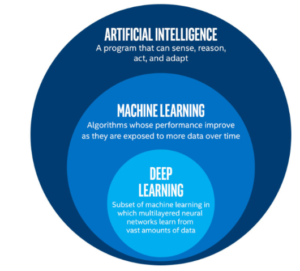
Machine Learning is a subset of AI which allows a machine to automatically learn from past data without programming explicitly. In other words, Machine learning (ML) is a branch of AI, as defined by the computer user and machine learning pioneer Tom M. Mitchell.
Tom M. Mitchell said, “Machine learning is the study of computer algorithms that allow computer programs to automatically improve through experience.”. ML is one of the ways we expect to attain AI. Click here to know that how M. Mitchell explained Machine Learning.
Machine learning
Machine learning relies on working with small to large datasets. By examining and comparing the info to seek out common patterns and explore nuances. In a simple example, if you load a machine learning program with a substantial large dataset of x-ray pictures with their description it will possess the capacity to help the information analysis of the x-ray pictures.
The machine learning model looks at each picture within the diverse dataset and finds common patterns. You will find these patterns in pictures with labels with comparable indications. Furthermore (assuming that we use a suitable ML algorithm for images), you load the model with new pictures. It compares its parameters with the previous examples to disclose how likely the images contain any of the previously anatomized indications.
Supervised Learning-a type of Machine Learning
Supervised learning is the type of machine learning where learning algorithms try and model relationships and dependencies between the target prediction output. Therefore the input features predict the output values for brand new data supported by those relationships which we learn from previous datasets. Unsupervised learning, another sort of machine learning, is that of the family of machine learning algorithms, which have main uses in pattern detection and descriptive modeling. These algorithms don’t have output categories or labels on the information (the model trains with labelless data).
Reinforcement learning- a type of Machine Learning
Reinforcement learning, the third popular style of machine learning. It uses observations gathered from the interaction with its environment to require actions that may maximize the reward or minimize the chance. This reinforcement learning algorithm (called the agent) continuously learns from its environment using iteration. An excellent example of reinforcement learning is computers reaching a super-human state and beating humans on computer games.
Machine learning is often dazzling, particularly its advanced sub-branches, i.e., deep learning and therefore the various sorts of neural networks. In any case, its “magic” (Computational Learning Theory) has issues observing its internal workings. Some tend to compare deep learning and neural networks with the way the human brain works but there are essential differences between the two.
Artificial intelligence (AI)
Artificial intelligence, on the opposite hand, is vast in scope. Andrew Moore, former Dean, College of Engineering Science at Carnegie Mellon University said, “AI is the science and engineering of creating computers to behave in ways in which we thought required human intelligence.” Fifty years ago, a chess-playing program was considered a type of AI since the theory of games and game strategies were capabilities that only someone’s brain could perform. Today, chess is dull and antiquated since it’s a part of almost every computer’s package (OS).
AI also includes a substantial measure of technological advances that we all know. Machine learning is barely in every one of them. Prior works of AI utilized different techniques i.e., Deep Blue, the AI that defeated the world’s chess champion in 1997. It used a technique called tree search algorithms to gauge a lot of moves at every turn. In today’s world, AI synchronizes with human-AI interaction such as gadgets by Google Home, Siri, Alexa, and by the machine-learning-powered video prediction systems that power Netflix, Amazon, and YouTube.
These technological advancements are progressively gaining more importance in our daily lives. They’re intelligent assistants who enhance our abilities as humans and professionals — making us more productive. In contrast to machine learning, AI is rapidly evolving so its definition changes as its related technological advancements advance. Shortly, today’s innovative AI advancements will be considered as dull as flip phones are to us today. Click here to read the complete blog of Artificial Intelligence Meaning.
In this blog, we tried to give you a synopsis of these two technology giants. Our mission is to help advance those communities that are far behind these technological achievements.